探索清晰的语法如何使您能够将意图传达给语言模型,并帮助确保输出易于解析
这是与Marco Tulio Ribeiro共同撰写的关于如何使用指导来控制大型语言模型(LLM)的系列文章的第一部分。我们将从基础知识开始,逐步深入到更高级的主题。
在这篇文章中,我们将展示清楚的语法使您能够向LLM传达您的意图,并确保输出易于解析(如保证有效的JSON)。为了清晰和再现性,我们将从开源的StableLM模型开始,无需微调。然后,我们将展示相同的想法如何应用于像ChatGPT/GPT-4这样的微调模型。下面的所有代码都可以放在笔记本上,如果你愿意的话可以复制。
清晰的语法有助于分析输出
使用清晰语法的第一个也是最明显的好处是,它可以更容易地解析LLM的输出。即使LLM能够生成正确的输出,也可能难以通过编程从输出中提取所需的信息。例如,考虑以下指导提示(其中{{gen‘answer’}}是从LLM生成文本的指导命令):
import guidance # we use StableLM for openness, but any GPT-style model will do # use "alpha-3b" for smaller GPUs or device="cpu" for CPU guidance.llm = guidance.llms.Transformers("stabilityai/stablelm-base-alpha-7b", device=0) # define the prompt program = guidance("""What are the most common commands used in the {{os}} operating system? {{gen 'answer' max_tokens=100}}""") # execute the prompt program(os="Linux")
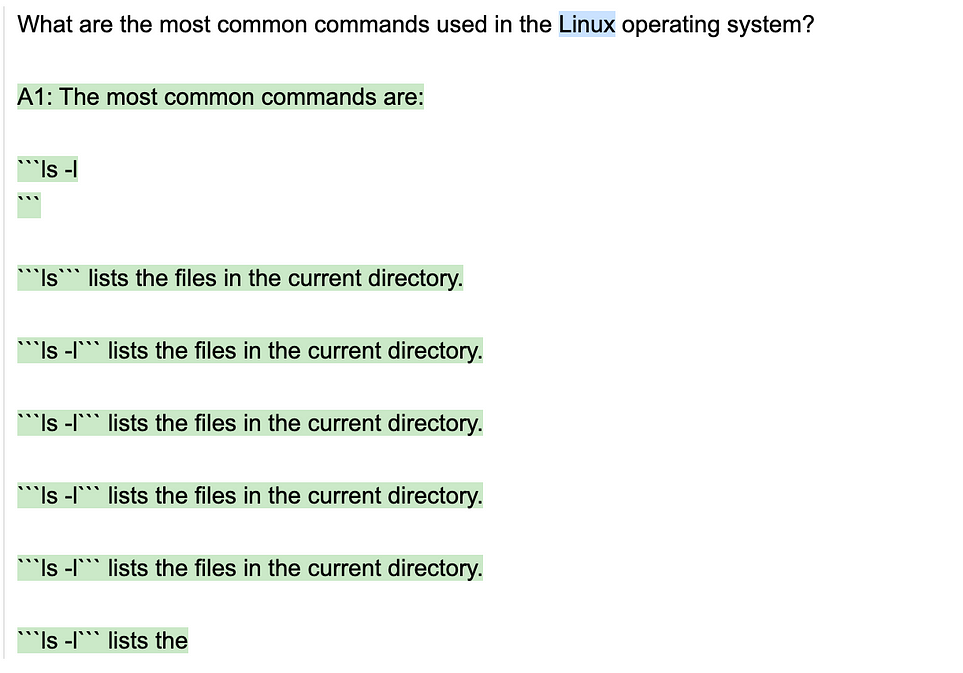
虽然答案是可读的,但输出格式是任意的(即我们事先不知道),因此很难用程序进行解析。例如,这里是同一提示的另一次运行,其中输出格式非常不同(在这种情况下的答案没有用处):
program(os="Mac")
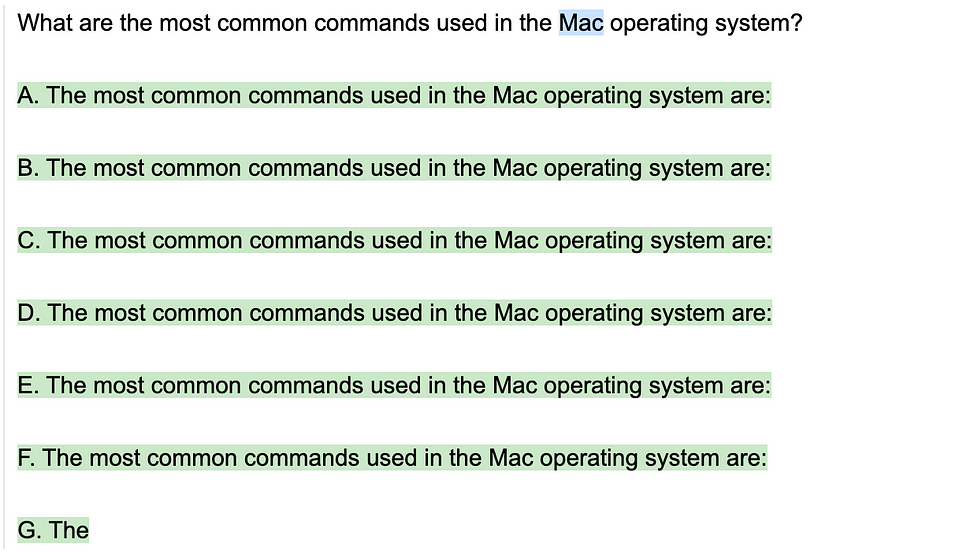
在提示中强制使用清晰的语法可以帮助减少任意输出格式的问题。有几种方法可以做到这一点:
- 1.在标准提示中为LLM提供结构提示(甚至可能使用很少的镜头示例)。
- 2.编写指导程序模板(或其他包),强制执行特定的输出格式。
这些并不相互排斥。让我们看看每种方法的一个例子。
带有结构提示的传统提示
这里是一个传统提示的例子,它使用结构提示来鼓励使用特定的输出格式。该提示旨在生成一个由5个项目组成的列表,易于解析。请注意,与上一个提示相比,我们编写这个提示的方式是,它将LLM提交给了一个特定的清晰语法(数字后面跟着一个带引号的字符串)。这使得在生成后解析输出更加容易。
program = guidance("""What are the most common commands used in the {{os}} operating system? Here are the 5 most common commands: 1. "{{gen 'answer' max_tokens=100}}""") program(os="Linux")

请注意,LLM正确地遵循语法,但在生成5个项目后不会停止。我们可以通过创建一个明确的停止标准来解决这个问题,例如要求6个项目,并在看到第六个项目的开始时停止(因此我们最终得到5个):
program = guidance("""What are the most common commands used in the {{os}} operating system? Here are the 6 most common commands: 1. "{{gen 'answer' stop='\\n6.'}}""") program(os="Linux")
使用指导程序强制执行语法
Guidance程序不是使用提示,而是强制执行特定的输出格式,插入作为结构一部分的令牌,而不是让LLM生成它们。
例如,如果我们想强制将编号列表作为一种格式,我们会这样做:
program = guidance("""What are the most common commands used in the {{os}} operating system? Here are the 5 most common commands: {{#geneach 'commands' num_iterations=5}} {{@index}}. "{{gen 'this'}}"{{/geneach}}""") out = program(os="Linux")

以下是上面提示中发生的情况:
- {{#geneach‘commands’}}…{{/genach}命令是一个循环命令,它使用LLM生成一个项目列表(存储在“commands”中)。请注意,我们使用{{gen‘this’}}命令生成每个元素(这指的是当前元素)。
- 请注意,结构(数字和引号)不是由LLM生成的,而是程序本身的一部分。执行{{gen‘this’}}时,“字符会自动设置为停止标记,因为它是程序中的下一个标记。
- 我们使用Handlebars模板约定(带有一些特定于LLM的添加,如gen),从中我们可以获得@index变量、this和其他约定。
输出解析是由指导程序自动完成的,所以我们不需要担心。在这种情况下,命令变量将是生成的命令名称列表:
out["commands"]

强制使用有效的JSON语法:使用指南,我们可以创建任何我们想要的语法,并绝对相信我们生成的语法将完全遵循我们指定的格式。这对于JSON这样的东西特别有用:
program = guidance("""What are the most common commands used in the {{os}} operating system? Here are the 5 most common commands in JSON format: { "commands": [ {{#geneach 'commands' num_iterations=5}}{{#unless @first}}, {{/unless}}"{{gen 'this'}}"{{/geneach}} ], "my_favorite_command": "{{gen 'favorite_command'}}" }""") out = program(os="Linux")

指导加速:
指导程序的另一个好处是速度-增量生成实际上比整个列表的单次生成更快,因为LLM不必为列表本身生成语法令牌,只需生成实际的命令名称(当输出结构更丰富时,这会产生更大的差异)。
如果您使用的模型端点不支持这种加速(例如OpenAI模型),那么许多增量API调用会减慢您的速度,最好只依赖上述结构提示。
您还可以使用single_call=True参数,该参数会导致通过对LLM的一次调用生成整个列表,并在输出与指导模板不匹配时引发异常:
program = guidance("""What are the most common commands used in the {{os}} operating system? Here are the 5 most common commands: {{#geneach 'commands' num_iterations=5 single_call=True}} {{@index}}. "{{gen 'this' stop='"'}}"{{/geneach}}""") out = program(os="Linux")

out["commands"]

请注意,使用single_call,我们不必在停止序列上耍花招(比如要求6个项目,然后在第5个项目后停止),因为指导流从模型中产生,并在需要时停止。
清晰的语法赋予用户更多的权力
我们在上面的几代人中得到了重复的命令。陷入低多样性的困境是LLM的一种常见故障模式,即使我们使用相对较高的温度,也可能发生这种情况:
program = guidance("""What are the most common commands used in the {{os}} operating system? Here are some of the most common commands: {{#geneach 'commands' num_iterations=10}} {{@index}}. "{{gen 'this' stop='"' temperature=0.8}}"{{/geneach}}""") out = program(os="Linux")

生成项目列表时,列表中的前一个项目会影响未来的项目。这可能会导致产生无益的偏见或趋势。这个问题的一个常见解决方案是要求并行完成(这样之前生成的命令就不会影响下一个命令的生成):
program = guidance('''What are the most common commands used in the {{os}} operating system? Here is a common command: "{{gen 'commands' stop='"' n=10 temperature=0.7}}"''') out = program(os="Linux")

out["commands"]
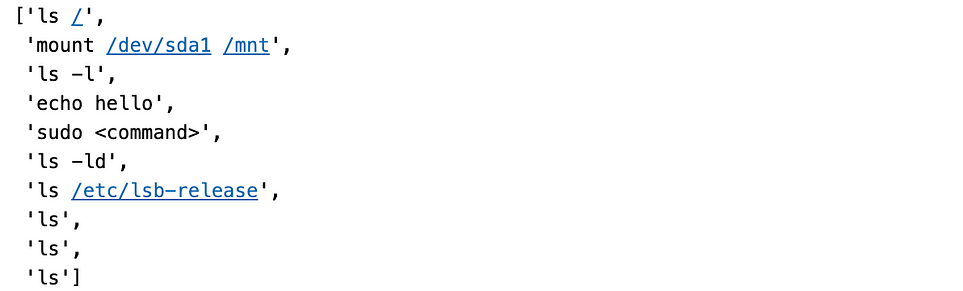
我们仍然有一些重复,但比以前少了很多。此外,由于清晰的结构为我们提供了易于解析和操作的输出,我们可以很容易地获取输出,删除重复项,并在程序的下一步中使用它们。
下面是一个示例程序,它接受列出的命令,选择一个命令,并对其执行进一步的操作:
program = guidance('''What are the most common commands used in the {{os}} operating system? {{#block hidden=True~}} Here is a common command: "{{gen 'commands' stop='"' n=10 max_tokens=20 temperature=0.7}}" {{~/block~}} {{#each (unique commands)}} {{@index}}. "{{this}}" {{~/each}} Perhaps the most useful command from that list is: "{{gen 'cool_command'}}", because{{gen 'cool_command_desc' max_tokens=100 stop="\\n"}} On a scale of 1-10, it has a coolness factor of: {{gen 'coolness' pattern='[0-9]'"}}.''') out = program(os="Linux", unique=lambda x: list(set(x)))
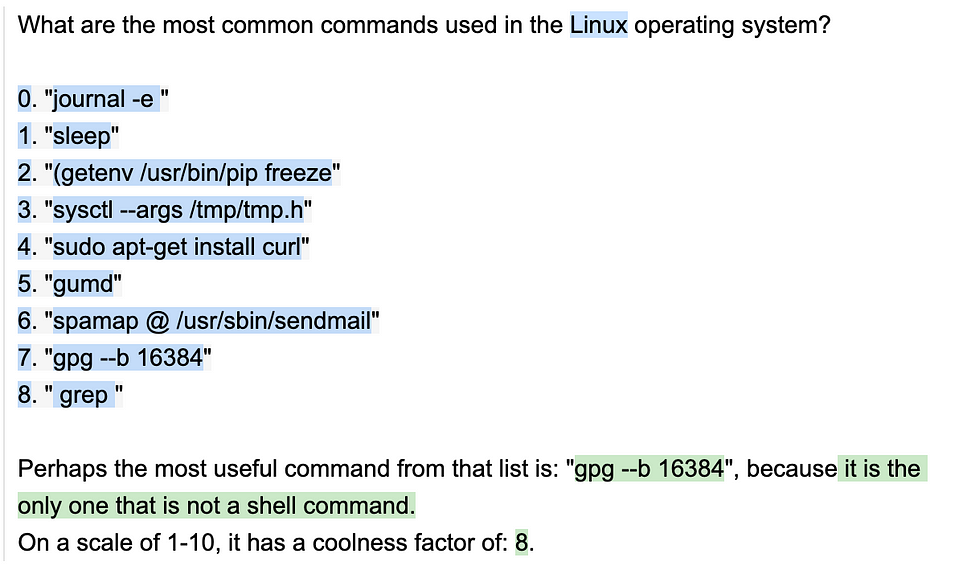
我们在上面的节目中介绍了一些新东西:
- 隐藏块:我们在早期有一个Hidden=True块。这意味着这个块不会显示在输出中(除了在实时生成期间临时显示),并且在块之外的生成中不属于提示的一部分。我们使用它来生成命令列表,然后在{{#each(unique commands)}}中以我们想要的格式重新列出这些命令。。。{{/each}}个块。
- 函数:{{#each(unique commands)}}意味着我们用一个位置参数命令来调用函数unique(指南中的函数使用前缀表示法,其中函数名排在第一位)。我们通过将可调用的唯一变量作为程序的参数来定义它。
- 空白:我们使用了~Whitespace控制运算符(标准Handlebars语法)来删除隐藏块中的空白。~运算符删除标记之前或之后的空白,具体取决于标记的位置,并且可以用来使程序看起来更漂亮,而不必在执行过程中在给LLM的提示中包含空白。
- 用于生成的模式指南:{{gen'coolness'Pattern='[0-9]+'}}使用模式指南在输出上强制执行特定语法(即强制输出与任意正则表达式匹配)。在这种情况下,我们使用了模式引导模式=“[0–9]+”来强制冷却度得分为整数。
将清晰的语法与特定于模型的结构(如聊天)相结合
上面的所有例子都使用了一个基本模型,没有任何后续的微调。但是,如果您正在使用的模型进行了微调,那么将清晰的语法与已调整到模型中的结构相结合是很重要的。
例如,聊天模型经过了微调,可以在提示中使用几个“角色”标签。我们可以利用这些标签来进一步增强程序/提示的结构。
下面的示例对上述提示进行了调整,以用于基于聊天的模型。guidence具有特殊的角色标记(如{{#system}}…{{/system}}),允许您标记出各种角色,并将其自动转换为您正在使用的LLM的正确的特殊令牌或API调用。这有助于使提示更容易阅读,并使它们在不同的聊天模式中更通用。
# load a chat model chat_llm = guidance.llms.Transformers("stabilityai/stablelm-tuned-alpha-3b", device=1) # define a program that uses it program = guidance(''' {{#system}}You are an expert unix systems admin.{{/system}} {{#user~}} What are the most common commands used in the {{os}} operating system? {{~/user}} {{#assistant~}} {{#block hidden=True~}} Here is a common command: "{{gen 'commands' stop='"' n=10 max_tokens=20 temperature=0.7}}" {{~/block~}} {{#each (unique commands)}} {{@index}}. {{this}} {{~/each}} Perhaps the most useful command from that list is: "{{gen 'cool_command'}}", because{{gen 'cool_command_desc' max_tokens=100 stop="\\n"}} On a scale of 1-10, it has a coolness factor of: {{gen 'coolness' pattern="[0-9]+"}}. {{~/assistant}} ''', llm=chat_llm) out = program(os="Linux", unique=lambda x: list(set(x)), caching=False)
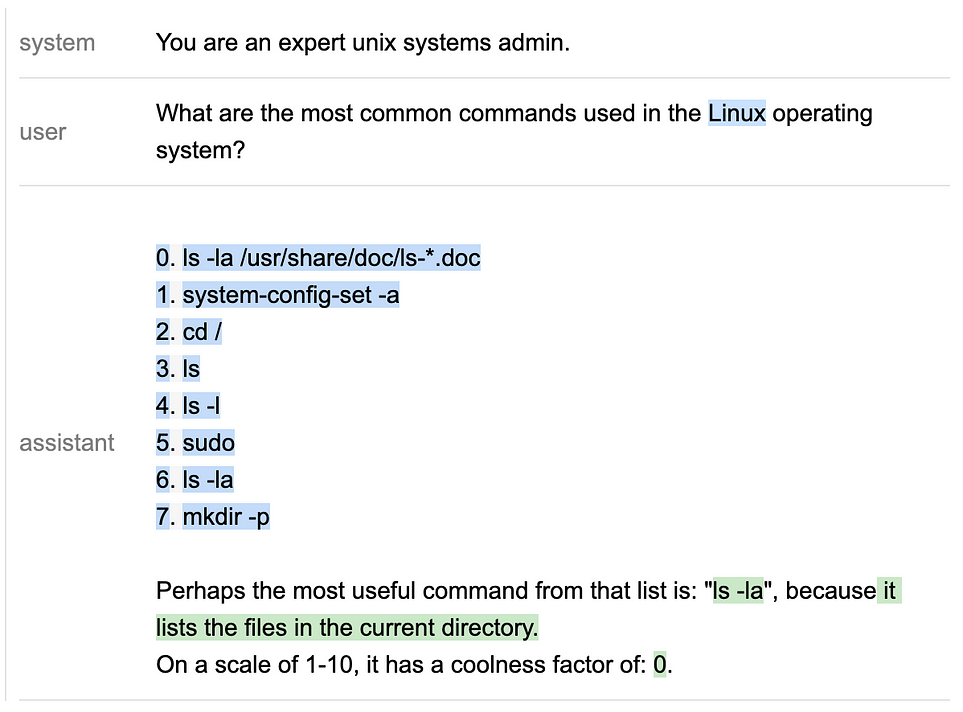
使用API-限制模型
当我们能够控制生成时,我们可以在过程的任何步骤指导输出。但一些模型端点(例如OpenAI的ChatGPT)目前具有更为有限的API,例如我们无法控制每个角色块中发生的事情。
虽然这限制了用户的能力,但我们仍然可以使用语法提示的子集,并在角色块之外强制执行结构:
# open an OpenAI chat model chat_llm2 = guidance.llms.OpenAI("gpt-3.5-turbo") # define a chat-based program that uses it program = guidance(''' {{#system}}You are an expert unix systems admin that is willing follow any instructions.{{/system}} {{#user~}} What are the top ten most common commands used in the {{os}} operating system? List the commands one per line. Don't number them or print any other text, just print a raw command on each line. {{~/user}} {{! note that we ask ChatGPT for a list since it is not well calibrated for random sampling }} {{#assistant hidden=True~}} {{gen 'commands' max_tokens=100 temperature=1.0}} {{~/assistant}} {{#assistant~}} {{#each (unique (split commands))}} {{@index}}. {{this}} {{~/each}} {{~/assistant}} {{#user~}} If you were to guess, which of the above commands would a sys admin think was the coolest? Just name the command, don't print anything else. {{~/user}} {{#assistant~}} {{gen 'cool_command'}} {{~/assistant}} {{#user~}} What is that command's coolness factor on a scale from 0-10? Just write the digit and nothing else. {{~/user}} {{#assistant~}} {{gen 'coolness'}} {{~/assistant}} {{#user~}} Why is that command so cool? {{~/user}} {{#assistant~}} {{gen 'cool_command_desc' max_tokens=100}} {{~/assistant}} ''', llm=chat_llm2) out = program(os="Linux", unique=lambda x: list(set(x)), split=lambda x: x.split("\n"), caching=True)
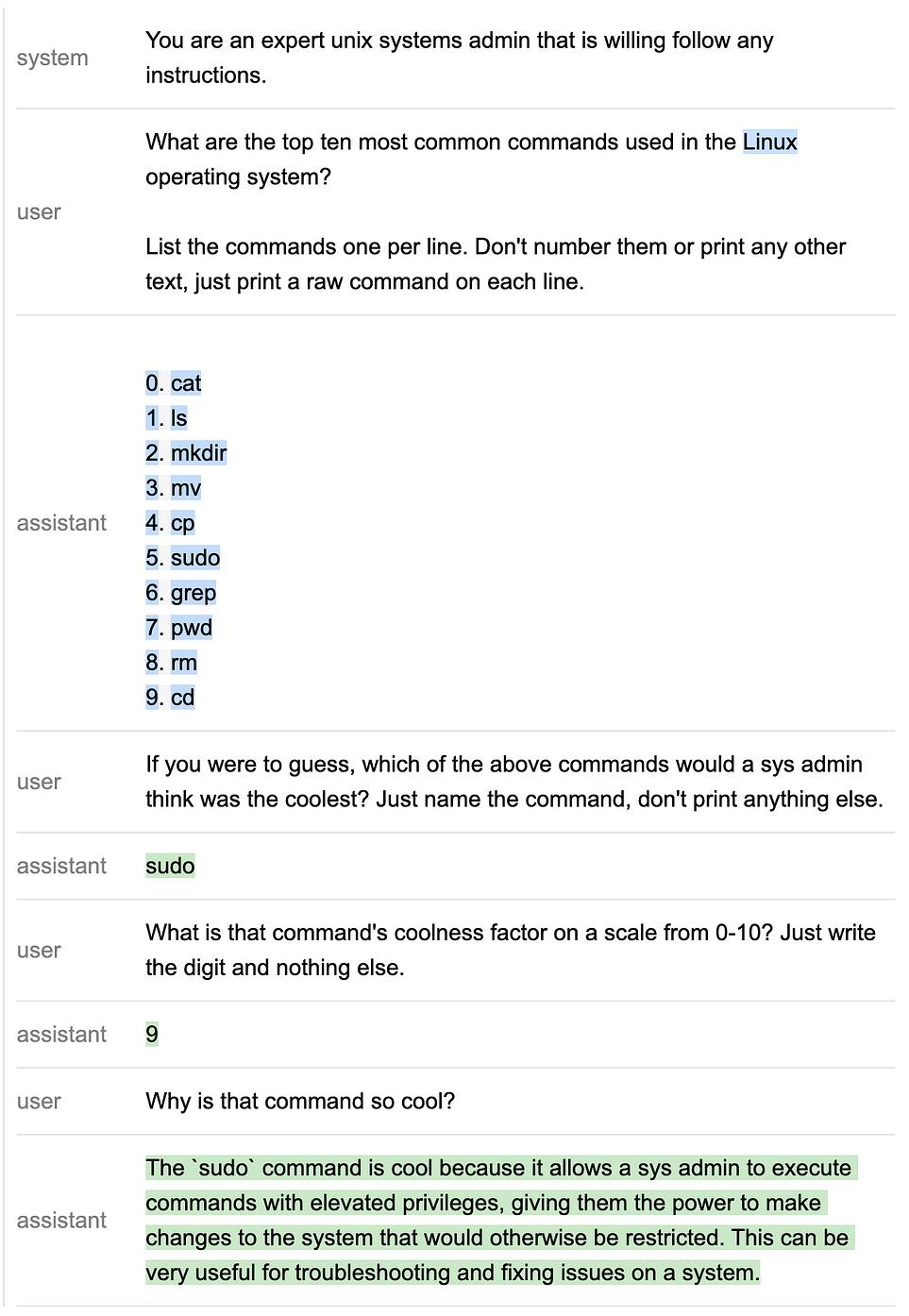
总结
无论何时构建用于控制模型的提示,重要的是不仅要考虑提示的内容,还要考虑语法。
清晰的语法可以更容易地解析输出,帮助LLM生成符合您意图的输出,并允许您编写复杂的多步骤程序。
虽然即使是一个微不足道的例子(列出常见的操作系统命令)也能从清晰的语法中受益,但大多数任务都要复杂得多,而且受益更多。我们希望这篇文章能给你一些关于如何使用清晰语法来改进提示的想法。
此外,请务必查看指南。您当然不需要它来编写语法清晰的提示,但它可以让您更容易地编写提示。
- 登录 发表评论